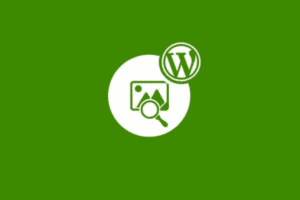
Image search for WordPress & Woocommerce
Image search is becoming more and more common with the advent of AI. It is very useful for many purposes but one of the most important is e-commerce. Using image search, users can upload images to find products that closely resemble what they are looking for, yielding far better conversion rates than text-based search ever would. So what if you wanted to add image search to Woocommerce, the world’s leading solution when it comes to e-commerce platforms. Learn all there is to know about WordPress search by reading our guide. How does image search work ? You can’t add image search to your WordPress or Woocommerce using the default search. The default WordPress search uses basic SQL to search for matching items